Chances are you’re working on an AI project for your 2025 plan. And if you’re exploring AI, you’ve got to be sure your data is in order – good AI needs great data. But how do you budget for an area that’s brand new? And how do you tell the story in a way that helps senior decision makers understand and support your projects in territory that is unfamiliar?
The good news is that the tools are more powerful and less expensive than ever, and the Data and AI category is becoming more mainstream. Despite this, getting funding for AI and data initiatives is far from a slam dunk. This blog outlines strategies to effectively scope and budget for the data portion of your AI project and build a strong path to approval.
Understanding Data Needs
The first step is to identify the data that AI will use. Cast a broad net, one of the beauties of AI is its ability to iterate quickly from the initial concept. Then conduct a thorough assessment to pinpoint critical areas that require data enrichment or improvement. This step sets the scope and defines the data you will need to access or acquire for your AI tools. It also ensures you get the right data.
Addressing Data Quality Issues
Poor data quality can significantly hinder the effectiveness of data and AI projects. Allocate resources to clean, validate, and standardize your data, ensuring its reliability and accuracy. Investing in data quality initiatives upfront will save time and effort in the long run. Tap your tech resources, especially data specialists, for insight and analysis. This step ensures you get the data right.
Prioritizing Data Initiatives
Not all data needs are created equal. Prioritize based on their alignment with business objectives, potential return on investment, and the urgency of addressing data-related challenges. A simple matrix of impact vs. level of effort (direct cost plus labor) is a good place to start. This will help you allocate resources effectively and respond quickly if you need to cut budget.
Leveraging Existing Infrastructure
Before investing in new infrastructure, assess your organization’s existing data resources. Identify opportunities to reuse or optimize existing systems, which can help reduce costs and accelerate project timelines.
Building a Strong Business Case
To secure internal funding and buy-in, develop a compelling business case that clearly articulates the project’s objectives, benefits, and expected outcomes. The best business cases are focused on the problems you are solving, and they tell a story that blends customer and team impact with data and metrics, to demonstrate the potential return on investment.
Fostering Collaboration and Alignment
Ensure that your data and AI projects are aligned with the organization’s overall strategy, and piggyback on existing priorities and funded programs. Collaborate with key stakeholders to build consensus, secure support, and avoid potential conflicts.
Budgeting for Data and AI Projects
Once you’ve defined your goals, it’s time to create a detailed budget. The data budget can be part of the AI project budget, or it can stand alone. Consider the following factors:
- Direct Technology Costs: Estimate the cost of necessary hardware, software licenses, and cloud services.
- Personnel Expenses: Calculate the salaries and benefits of data scientists, data engineers, and other team members involved in the project.
- Data Acquisition and Preparation Costs: Factor in the expenses of acquiring, cleaning, and preparing data for analysis.
- Ongoing Maintenance and Support: Consider the ongoing expenses for maintaining and supporting your data and AI infrastructure.
To prove ROI year over year, establish key performance indicators (KPIs) that align with your project’s objectives. Track these metrics regularly and compare them to the budgeted costs. This will help you assess the project’s effectiveness and make informed decisions about future investments. Additionally, consider conducting cost-benefit analyses to quantify the financial benefits of your data and AI initiatives. By demonstrating a positive ROI, you can justify continued funding and support for your projects.
Additional Considerations
- Data Governance: Be sure you comply with your organization’s data governance policies to ensure data security, privacy, and compliance with regulations.
- Talent Acquisition and Development: Consider adding staff or hiring consultants If you don’t have access to internal specialists, such as data scientists, and data engineers.
- Ethical Considerations: Address ethical implications related to data usage, bias, and transparency in AI applications.
Considering the data factors and implementing effective budgeting strategies helps maximize the value of your AI investments and drive sustainable growth.
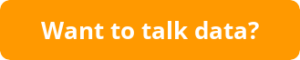